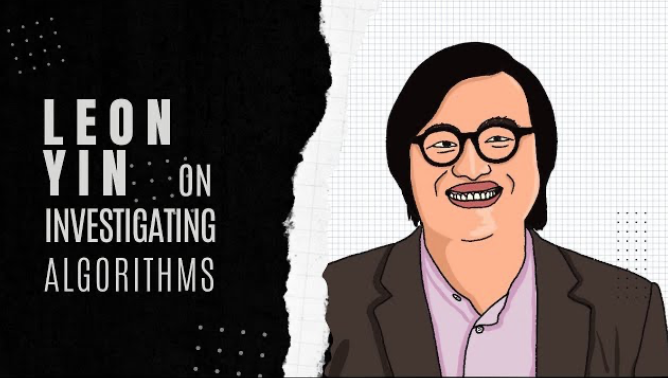
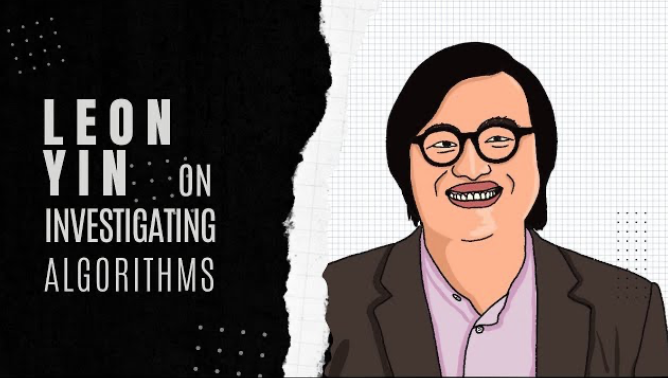
Bloomberg Technology investigative data journalist Leon Yin has trained his reporting focus on how major social networks' algorithms function. Image: Screenshot, In Old News, YouTube
Unlocking the Secrets of Algorithms: Q & A with Investigative Data Journalist Leon Yin
Read this article in
Leon Yin is an investigative data journalist recognized for his work on how technology impacts society. He worked on the investigation that uncovered how Google doesn’t allow advertisers to use racial justice terms like Black Lives Matter while allowing the term White Lives Matter. Another investigation found that Facebook hasn’t been transparent about the popularity of right-wing content on its platform. And he worked on a project that uncovered that Google search prioritizes its own products in the top search results over other products. This work was mentioned in the antitrust hearings in July 2020 held by a US Congressional subcommittee.
When we interviewed Leon at the International Journalism Festival, he was working at The Markup, a nonprofit newsroom focused on being the watchdog of the tech industry. He has since joined Bloomberg Technology, where he’s continuing his investigative work.
In addition to his articles, Leon publishes resources that explain how he worked on his investigations. Among the resources is Inspect Element, a guide he put together on how to conduct investigations into algorithms, build datasets, and more.
In Old News: Are you usually working on several projects at once? How do you balance that with learning new skills?
Leon Yin: I say that I tend to like to work on two stories at once that are long term or like if something slows down, I can move to something else, either another long-term something or something with a quicker churn, just to keep moving. Because it’s easy to stagnate sometimes. I think that a lot of times with these investigations, you really just need time, and you need review from others and feedback.
ION: One of your stories was mentioned during a congressional hearing. Could you walk us through how that happened, and is that an outcome you were expecting?
LY: The way that started was my reporting partner, Adrianne Jeffries — she’s been reporting on Google for ages and she had done a lot on the featured snippet, which is the text box that’s been scraped from the web and summarizes something. She’s really fascinated about [that].
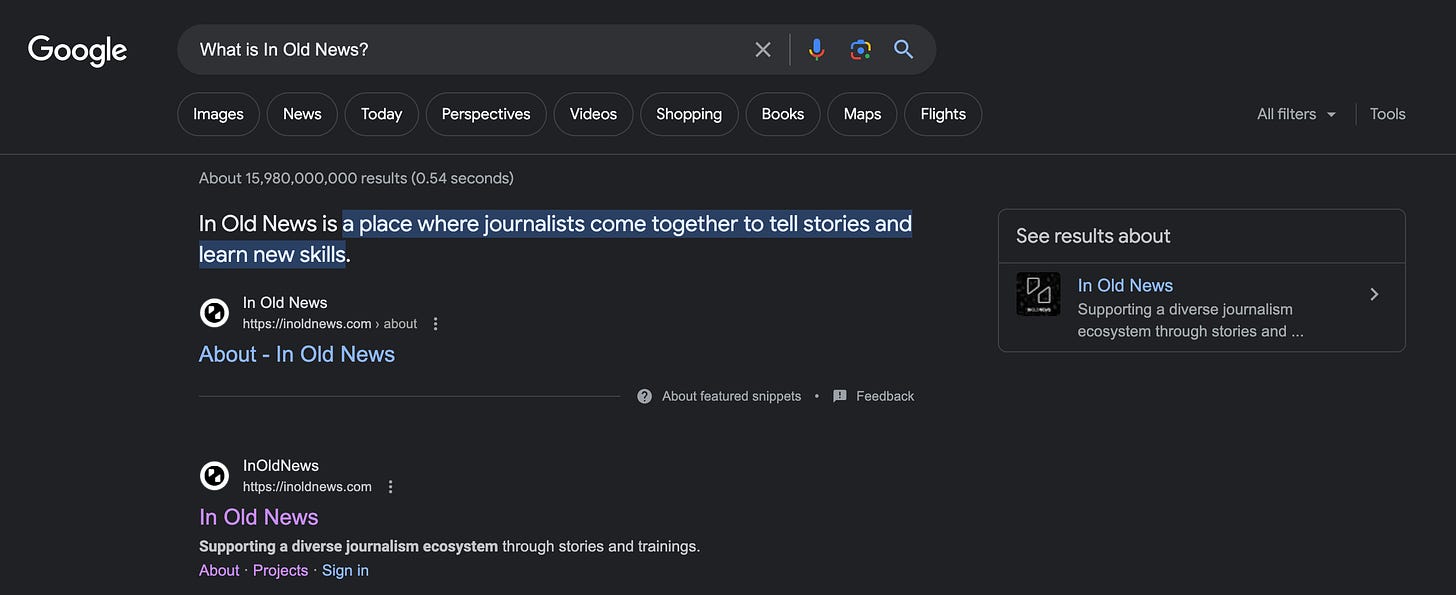
Example of a featured snippet in Google search. Image: Screenshot, Google
We also developed this way of actually staining the web page and getting the real estate, because even though we could tell it was Google, we still need to measure something. And we ended up finding this way to selectively create bounding boxes around different elements of the page, some that were Google and some linked out and we colored a specific color, then took the sum of that color divided by the total real estate of the page and it ended up being roughly 42% of the first page was all to Google.
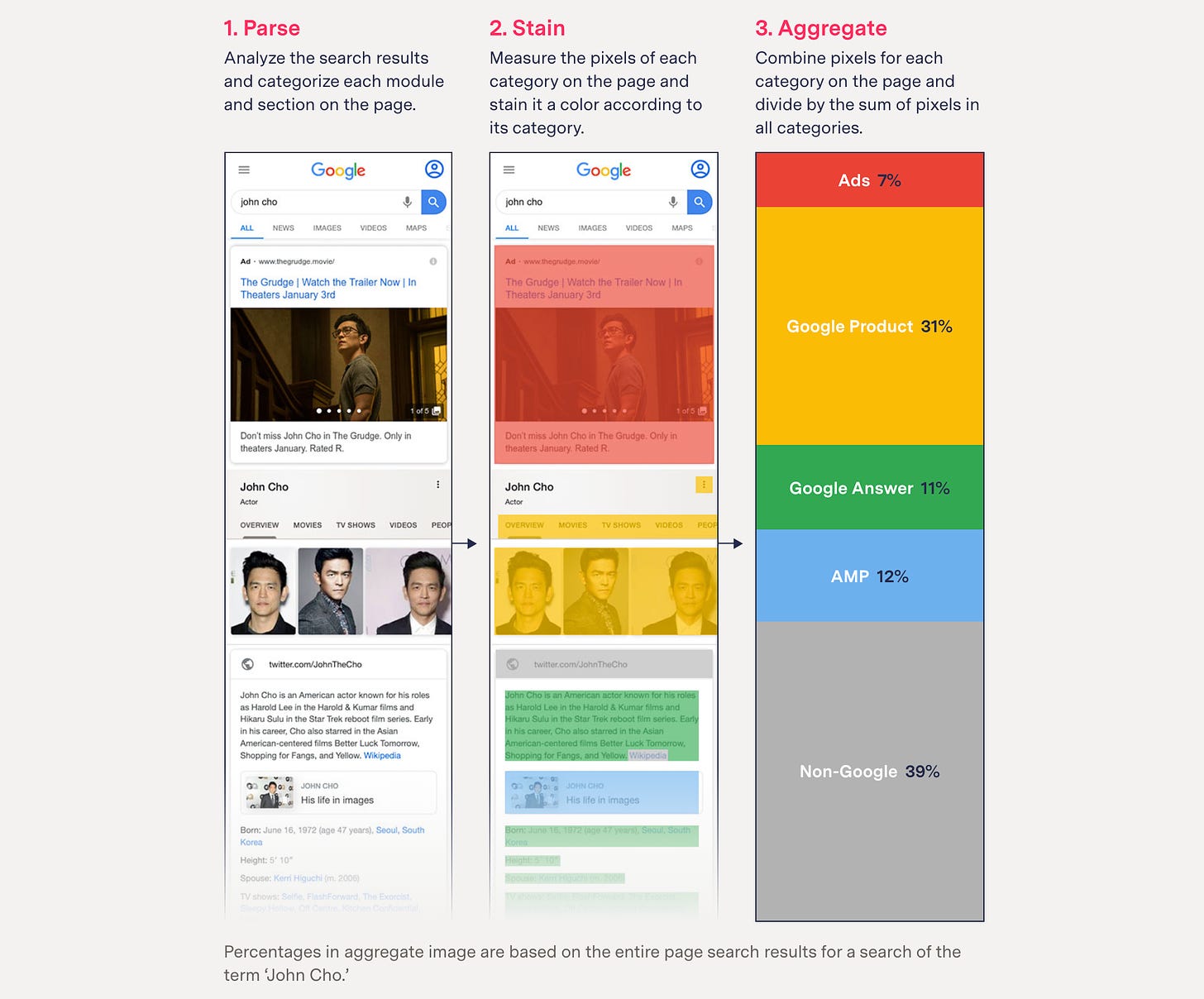
Image of the web staining technique Leon and his team used to investigate Google search results. Image: Screenshot, leonyin.org
That was a stat that we finally had about this theoretical stat that everyone kind of knows. Google self-preferences itself, it owns the bakery, it makes the bread, of course it’s going to be like, “check out our rolls,” right? But to actually find that number was really powerful. And so, I love doing that thing where there’s common knowledge but no evidence, right?
We go in and fill that void. So when that came out, there was also the Congressional hearing about antitrust, where all of the big tech CEOs were being questioned by Congress and in the opening remarks, I think [then-US Congressman] David Cicilline, brought up our work when they’re questioning Google. And it was just unbelievable. We’re like, my God, how’d this happen? So sometimes our work has this impact and we have no idea it’s going to happen or timing happens quite well. I don’t know if we went to his staff and shared those documents. I don’t know if we always do that. I don’t think we did in that case.
A very similar thing happened with our investigation of Amazon, which was a logical follow-up. How can we do this from another big tech platform that self-preferences itself?
We looked at Amazon private label products, popular Amazon searches, and within days of publication, which is pretty much a year later, [Congress] sent a letter to Amazon leadership [saying]: Under oath, you said that Amazon doesn’t preference its products, but this study says directly the opposite of that. Please explain.
It’s just amazing. When we pitch stories, we always think about that accountability angle. Like, what is the thing that’s being broken? Who’s being impacted? Somebody lied under oath and we can prove that. Or a law is being broken. So I feel that’s the way we frame our stories. Often it just so happens that that happened to be something that an executive said under oath.
We didn’t even know that at the time, but it happened. So that’s some of the impact we’ve had.
ION: How do you figure out if a resource-intensive story is viable?
LY: One thing that helps me and my editor determine if the story’s viable is this kind of checklist I mentioned before. One of the questions off the bat is can you test something with data? How would you get the data, how difficult it’s going to be? What do you have to classify, categorize? We always end up with having to ascribe a value to data because oftentimes the output variable that we’re looking for— like what’s Google, what’s Amazon — it’s not a neat column. We have to figure out what it is. So not only do we have to figure out what it is, we have to figure out what that dataset is, what that universe is like. Where do you stop? What’s enough? What’s a quick test to see if something’s viable?
I’m always thinking about these questions and trying to fill them out and approach my editor with as many as possible before we go fully in. Another thing that we do is a quick test — what’s the minimum viable analysis to prove that something is measurable, something’s afoot? To see if a pattern is available.
That’s actually how a recent story on internet disparities in the United States started, where we were trying to reproduce an academic article that had looked at a ton of different internet service providers in nine states. To do that, we looked at one provider, in one small city. I found there are pockets of fast speeds and slow speeds, which seemed kind of unfair, but also the prices were exactly the same if you’re getting fast speeds or slow speeds. And we joined census data and found that it was really skewed. Lower-income people were getting more slow speeds at the same price.
That quick test not only tells you the viability but whether you have a different story. And so we changed our story angle from being that academic study, which is more of a policy story, to a consumer side story of being: You’re getting a bad deal and it’s happening all across the country.
So within this checklist of questions that we ask, we also think: What’s a quick analysis you can do to show there’s something. That’s something I send my editor and then we discuss. It’s usually clear if you have a story or not. It’s really clear if you have a better story or not. And it’s also clear if you need to kill a story if you have just unanswerable questions of things that are just too uncertain.
ION: How important is it for journalists to show their work?
LY: I think the reason why [The Markup founder] Julia Angwin emphasized the necessity to do that is to build trust. To say that we’re not p-hacking, we’re not making shit up. This is the evidence. And you can see exactly how we got to our conclusions. You can draw your own conclusions.
I think there’s a lot of strength in that. I just keep going back to just traditional journalism. If you have documents or interviews, you would host them somewhere like Muck Rock or on DocumentCloud, right? To say, here’s my FOIA [Freedom of Information Act] request. Here is the information that I got from that request.
Fundamentally, it’s similar [to my work] where I’m making a request not to a government agency but to a website. Here’s what I asked them for, here’s the document that they gave me, and here’s what I did with that document. So I just see it building on traditional journalism. Just at a scale and using a different kind of language of doing so.
It all kind of goes back to the fundamentals. I think that I would love to see more newsrooms invest in this evidence creation of building their own data sets to answer questions. I have seen longer write-ups and methodologies become more popular. Consumer Reports, their investigative team does that. The Washington Post does that. So I’m starting to see more of a change, but it’s still a rarity. Whereas in most of the stories I work on, it’s a necessity. Because we want myself, my reporter, my editor, and whoever we investigate to understand that we have the information down. That we all know exactly what’s being measured, where it comes from, our assumptions, the limitations.
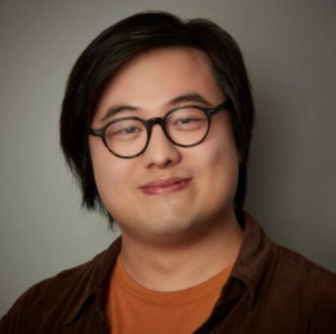
Leon Yin. Image: Screenshot, Twitter/X
It’s all emphatic and necessary to make bold claims that are accurate rather than making bold claims that are actually unsubstantiated by evidence, [where] it’s not clear how you got it or what test you ran. It’s all about the details, but making them really apparent. Of everything important to it to be solid. And we did nothing to exaggerate what you’re seeing. The clearest examples of disparities, of patterns, are so apparent. I feel like it’s our job just to put the data together, follow the pattern, anyone will find the same conclusion. That’s a skill that I’m hoping to impart on others.
I have a tutorial for a skill that I use a lot, which is finding undocumented APIs. APIs are how servers interact with the website and there are a lot of them that aren’t official APIs, but they still work on websites. It’s a subset of web scraping and like almost every story I work on is thanks to finding one of those. To me, data is just another thing you have to fact check. But not anyone is capable of doing that. That’s why I feel like that reviewing stuff is super important just to make sure that everything is accurate, reproducible, explainable. Hopefully those ways all land on the same answer. It assures you’re not cherry-picking and it assures that you’ve chosen a method that’s reasonable. Because we have these long methodologies, we can disclose that. To show that we tried another categorization scheme or we tried another model and the results are interpreted very similarly. Because our data is made public, others could do that too. So that’s a great strength of our work.
This post was originally published on the Substack site In Old News. It has been lightly edited for length and clarity and republished with permission. You can watch the YouTube video of this interview below.
In Old News aims to support a diverse journalism ecosystem through stories and trainings. The organization has trained thousands of journalists in over 65 newsrooms to use their phones for multimedia journalism with the aim of supporting a diverse journalism ecosystem through stories and trainings. It also publishes stories “of journalism from journalists.”