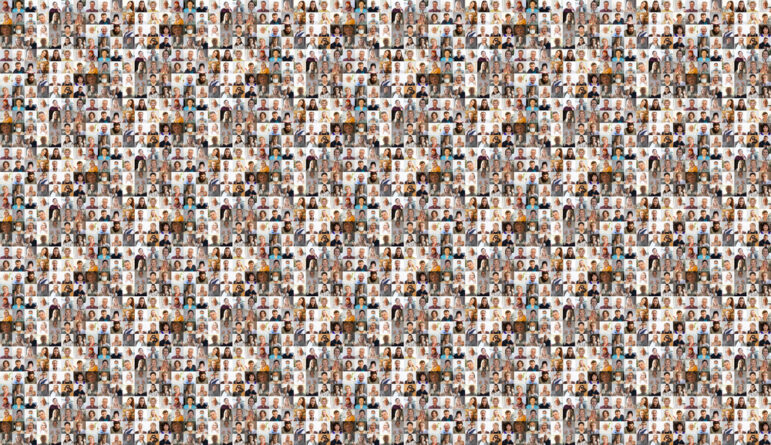
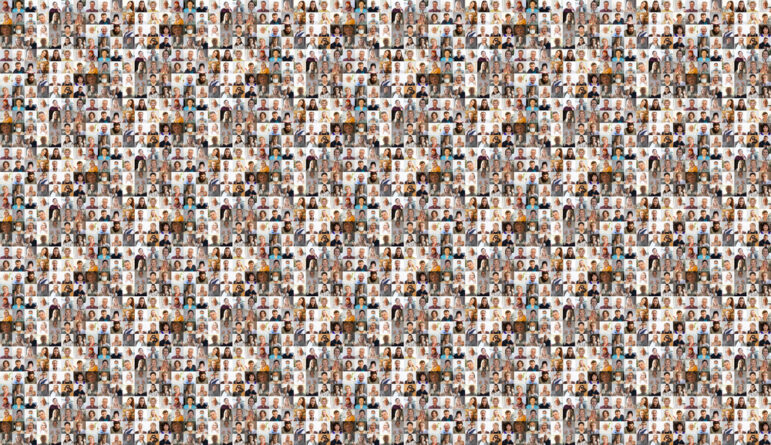
Tips for Investigating Algorithm Harm — and Avoiding AI Hype
Read this article in
In January, data scientist and journalist Gianluca Mauro decided to use himself as a test subject, to see how artificial intelligence-driven algorithms treat images on social media.
Posing shirtless in long pants, Mauro’s selfie photo received only a 22% score for “raciness” from a Microsoft classifier AI tool — meaning his image would likely be able to be promoted on social media posts.
By simply adding a bra to this same outfit, he found that the algorithm suddenly slammed his image with a 97% raciness score, which would see it severely suppressed.
Mauro’s broader investigation — written with freelance investigative reporter Hilke Schellmann — found shocking gender biases in many platform algorithms, with pregnant bellies, women’s underwear, and even important women’s health explainers suppressed by algorithms as “sexually suggestive,” while equivalent images of male features easily passed the AI-driven test for alleged appropriateness.
Meanwhile, journalists have found similarly blatant racial biases in other algorithms that cause deeper harms, from disparate child welfare decisions in the US and targeted raids on minorities in the Netherlands to inadequate housing allocation and surveillance of student dissent.
In a panel discussion of the topic produced in collaboration with the Pulitzer Center at IRE23 — the annual watchdog conference hosted by Investigative Reporters and Editors — Schellmann and two other experts shared tips on how reporters can identify algorithmic harms, and hold the human masters of these systems accountable.
One key takeaway from the panelists was that these investigations are about studying human decisions and community outcomes, rather than analyzing code.
“I wouldn’t know what to do with an actual algorithm if I saw one,” Schellmann quipped.
As a result, evidence such as email threads, human training inputs, victim interviews, and ordinary data journalism tend to be more important than technical efforts to reverse-engineer these complex systems.
Other speakers included Garance Burke — an investigative reporter at the Associated Press who specializes in AI technologies — and Sayash Kapoor, a computer science Ph.D. candidate at Princeton University’s Center for Information Technology Policy.
“There are multiple ways biases can seep into these technologies,” said Burke. “Whether you cover education or policing or politics, you can find specific AI-related stories in the public interest.”
Avoiding the Pitfalls of AI Hype
In a recent article for the Columbia Journalism Review, Schellmann, Kapoor, and Dallas Morning News reporter Ari Sen explained that AI “machine learning” systems are neither sentient nor independent. Instead, these systems differ from past computer models because, rather than following a set of digital rules, they can “recognize patterns in data.”
“While details vary, supervised learning tools are essentially all just computers learning patterns from labeled data,” they wrote. They warned that futuristic-sounding processes like “self-supervised learning” — a technique used by ChatGPT — do not denote independent thinking, but merely automated labeling.
So the data labels and annotations that train algorithms — a largely human-driven process that coaches the computer to find similar things — are a major source of questions for investigative reporters on this beat. Do the labels represent the whole population affected by the algorithm? Who entered those labels? Were they audited? Do the training labels embed historic discrimination? For instance, if you simply asked a basic hiring algorithm to evaluate job applicants for a long-standing engineering company, it would likely discriminate against female candidates, because the data it has for most prior hires would most likely overwhelmingly feature “male” labels.
Increasingly, government agencies and universities around the world spend vast sums for algorithmic tools — often, to generate automated scoring systems to streamline the allocation of benefits.
In addition to checking for bias and unintended harm within these systems, Kapoor urged reporters at IRE23 to also ask serious questions on whether they work at all for their stated purpose.
This turned out to be a major angle for Ari Sen’s 2022 Dallas Morning News investigation into an AI-driven social media monitoring service used by 38 colleges and hundreds of school districts around the US. In addition to discovering illicit student surveillance, Sen could find no evidence that this hugely expensive tool had fully worked for its stated purpose on even one occasion.
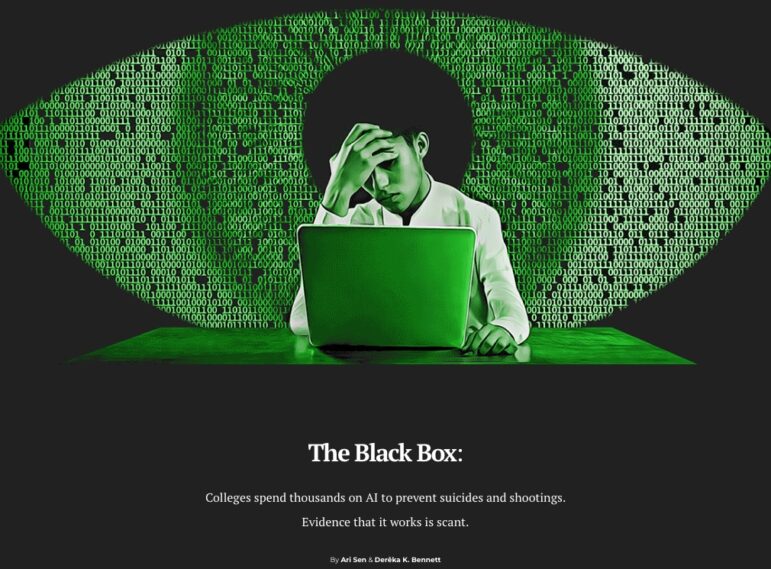
A Dallas Morning News investigation into colleges’ use of AI to prevent suicides and shootings found no data that the system worked and, instead, found troubling evidence of illicit secret surveillance of students. Image: Screenshot, Dallas Morning News
“One aspect missed by media is ‘Does this tool even work?,” explained Kapoor. “Some AI hiring tools, for instance, involve slapping on random number generators that are unable to distinguish positive work characteristics from negative ones.”
Kapoor warned that the subject of AI is so riddled with overhyped claims, misleading pop culture representations, and erroneous media statements that it is important for journalists to first check for conceptual errors before embarking on an investigation.
“Performance of AI systems is systematically exaggerated because of things like publication bias,” said Kapoor. “Public figures make tall claims about AI — they often want you to believe AI systems are God-like, and they are distracting from the real issues. There are conflicts of interest, bias, and accountability issues to watch. We should focus on near-term risks.”
In an analysis of 50 stories on AI and algorithms from major media, Kapoor and a colleague identified 18 “recurring pitfalls” that cause journalists to unwittingly mislead audiences on the subject.
Here is a rundown of experts’ tips for more clearly investigating algorithms:
- Since algorithms are often proprietary, look for tech vendors working with government agencies. “It’s tricky, because you can’t FOIA private companies, so you need to ask ‘Where do these companies interact with public entities?’” said Schellmann.
- Focus on emails. “Emails are really important to go after — they can show you the humans in this chain, those excited about this tech, and the government officials who want to spread this tool to the next level,” said Burke. “Emails may also show what assumptions humans have about the AI tools they are about to use,” Schellmann added.
- Test algorithms directly, yourself. Schellmann suggests that reporters benchmark algorithms that measure the same thing against each other, to see, for instance, how the results differ. She also suggests they test openAI classifier tools for potential biases across various metrics. In some cases, reporters can try signing up for trial accounts from vendors to test their algorithms. “Journalists can run the AI tools and then compare them to traditional personality tests,” Schellmann explained.
- Ask the “if-then” AI accountability question. “Say to the agency using the AI tool: ‘If you say the AI doesn’t make decisions, and that you do, then why would you pay hundreds of thousands of dollars for a tool that ranks people, and then ignore it?’” Schellmann suggested.
- Ask for audits of algorithm performance. But be cautious of corporate “whitewashing” and conflicts of interest in these reviews. “It’s important to look for audits and evaluations of the effectiveness of the tool for public agencies. They can contain interesting nuggets,” said Burke. “In Colorado, we found one audit that showed the mere existence of the tool made social workers something like 30% more likely to rank families as high risk [for children] — the kind of confirmation bias we need to worry about.”
- If you can’t get training label data, ask for the “variables” and “weights” selected for the algorithm. “We put out a lot of FOIA requests, and what we sometimes got were the variables chosen to build the algorithm — data points for what the tool is trying to predict — and, in instances, the weights assigned to those variables,” said Burke. “So, say, ‘How important does the algorithm think it is that you have five siblings in your family?’ If you can get those two things, you get a sense of the shape of the elephant, even if you don’t get all of the training data, which can be a long battle.”
- Check patent databases and, especially, trademark databases for new tools companies are developing. “Trademark is where companies often file stuff directly relevant to algorithms,” said Schellmann.
- Speak to lawyers and consultants in the arena. “Key sources of mine are attorneys, who get called in by companies to do some sort of due diligence, and so have some knowledge of these systems,” Schellmann added.
- Avoid images of humanoid robots. In one example of a media practice that can mislead audiences, Kapoor pointed to the pervasive use of humanoid robot images in stories about AI and algorithms, when, in fact, these systems generally “work more like a spreadsheet,” with no futuristic hardware involved at all.
- Challenge algorithm claims with crowdsourced data. Schellmann, however, warned that crowdsourcing should be a “last resort,” as this tactic is labor-intensive, and can involve serious logistical challenges, as Der Spiegel found in an investigation into Germany’s credit scoring system in 2019.
- Ask if the algorithm has been changed or updated — and why. If so, Schellmann said reporters should check to see if other customers are still using the earlier version of the same tool, and ask what errors and harms this could generate.
- Focus on people impacted by the technology. Burke suggested that reporters dig through the AP’s Tracked investigative series for tips on how to match algorithm rankings against human impacts.
- Interrogate the labeling process. Panelists said reporters should ask key questions of AI vendors: “Does the training data actually represent the population it’s being used on?” “Is the training dataset based on only a small sample, like 100 rows?” “Who is doing the labeling?” “Has the company ever had their training data blinded or audited?”
Said Burke: “If you are patient and do community-based reporting, you can find real impacts from these tools.”
Schellmann also encouraged reporters to push past any intimidation they might feel about the computer science aspect of algorithms. “I’m a freelance journalist, and I don’t even code, so anyone can do this stuff,” she noted. “It’s mostly journalists who have uncovered serious harms these tools have inflicted on incarcerated people, job applicants, people seeking social services, and many more. This seems to be the only way companies will change their flawed tools.”
Additional Resources
10 Things You Should Know About AI in Journalism
Testing the Potential of Using ChatGPT to Extract Data from PDFs
Journalists’ Guide to Using AI and Satellite Imagery for Storytelling
Rowan Philp is a senior reporter for GIJN. Rowan was formerly chief reporter for South Africa’s Sunday Times. As a foreign correspondent, he has reported on news, politics, corruption, and conflict from more than two dozen countries around the world.