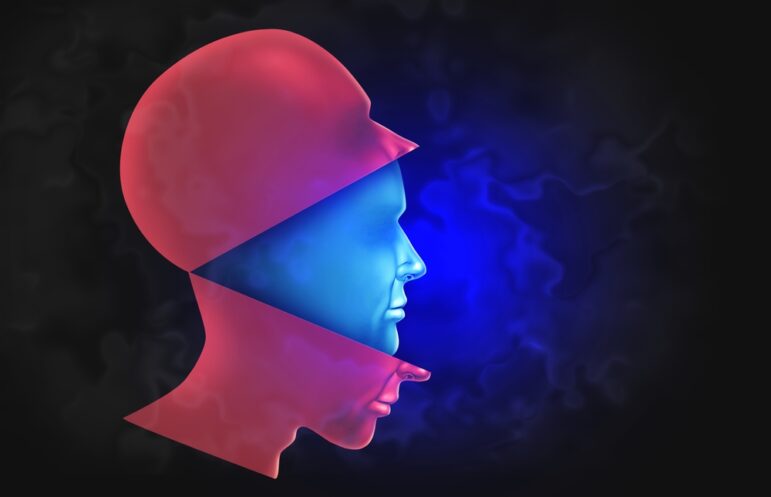
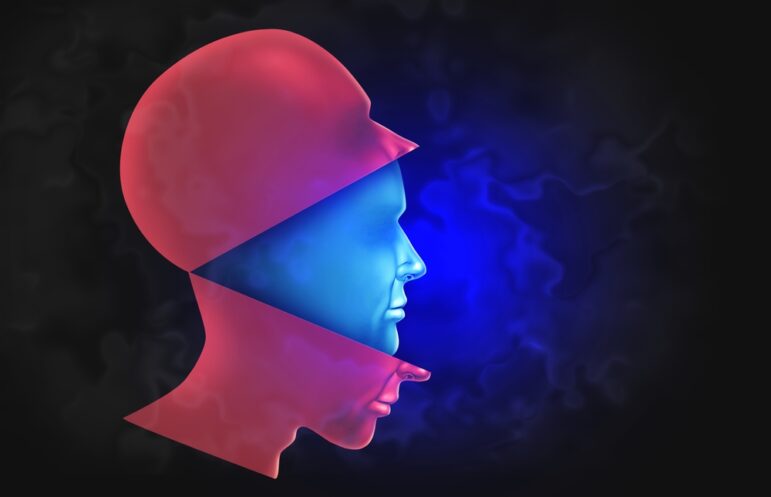
Image: Shutterstock
Spotting Deepfakes in an Election Year: How AI Detection Tools Work — and Sometimes Fail
Read this article in
With the progress of generative AI technologies, synthetic media is getting more realistic. Some of these outputs can still be recognized as AI-altered or AI-generated, but the quality we see today represents the lowest level of verisimilitude we can expect from these technologies moving forward.
We work for WITNESS, an organization that is addressing how transparency in AI production can help mitigate the increasing confusion and lack of trust in the information environment. However, disclosure techniques such as visible and invisible watermarking, digital fingerprinting, labelling, and embedded metadata still need more refinement to address at least issues with their resilience, interoperability, and adoption. WITNESS has also done extensive work about the socio-technical aspects of provenance and authenticity approaches that can help people identify real content. See, for instance, this report or these articles here and here.
A complementary approach to marking what is real is to spot what is fake. Simple visual cues, such as looking for anomalous hand features or unnatural blinking patterns in deepfake videos, are quickly outdated by ever-evolving techniques. This has led to a growing demand for AI detection tools that can determine whether a piece of audio and visual content has been generated or edited using AI without relying on external corroboration or context.
While not a perfect fit as a term, in this article we use “real” to refer to content that has not been generated or edited by AI. Yet it is crucial to note that the distinction between real and synthetic is increasingly blurring. This is due in part to the fact that many modern cameras already integrate AI functionalities to direct light and frame objects. For instance, iPhone features such as Portrait Mode, Smart HDR, Deep Fusion, and Night mode use AI to enhance photo quality. Android incorporates similar features and further options that allow for in-camera AI-editing.
This piece aims to offer preliminary insights on how to evaluate and understand the outcomes provided by publicly accessible detectors that are available for free or at low cost. Based on testing conducted in February 2024 of a sample of online detectors that included Optic, Hive Moderation, V7, Invid, Deepware Scanner, Illuminarty, DeepID and open-source AI image detector, we discuss where these tools may currently experience limitations, as well as what factors need to be considered when deciding to use them. It is important to acknowledge that, akin to generative technologies, the development of detection models is ongoing. Therefore, a tool’s performance may vary over time: it might struggle to accurately identify certain manipulations at one point but excel in detecting others as it evolves. This dynamic nature underscores the challenges in synthetic media detection tools and the necessity of staying informed about their latest advancements.
How Understandable Are the Results?
AI detection tools provide results that require informed interpretation, and this can easily mislead users. Computational detection tools could be a great starting point as part of a verification process, along with other open source techniques, often referred to as OSINT methods. This may include reverse image search, geolocation, or shadow analysis, among many others.
However, it is essential to note that detection tools should not be considered a one-stop solution and must be used with caution. We have seen how the use of publicly available software has led to confusion, especially when used without the right expertise to help interpret the results. Moreover, even when an AI-detection tool does not identify any signs of AI, this does not necessarily mean the content is not synthetic. And even when a piece of media is not synthetic, what is on the frame is always a curation of reality, or the content may have been staged.
Most of the results provided by AI detection tools give either a confidence interval or probabilistic determination (e.g. 85% human), whereas others only give a binary “yes/no” result. It can be challenging to interpret these results without knowing more about the detection model, such as what it was trained to detect, the dataset used for training, and when it was last updated. Unfortunately, most online detection tools do not provide sufficient information about their development, making it difficult to evaluate and trust the detector results and their significance.
How Accurate Are These Tools?
AI detection tools are computational and data-driven processes. These tools are trained on using specific datasets, including pairs of verified and synthetic content, to categorize media with varying degrees of certainty as either real or AI-generated. The accuracy of a tool depends on the quality, quantity, and type of training data used, as well as the algorithmic functions that it was designed for. For instance, a detection model may be able to spot AI-generated images, but may not be able to identify that a video is a deepfake created from swapping people’s faces.
Similarly, a model trained on a dataset of public figures and politicians may be able to identify a deepfake of Ukraine President Volodymyr Zelensky, but may falter with less public figures like a journalist who lacks a substantial online footprint.
Additionally, detection accuracy may diminish in scenarios involving audio content marred by background noise or overlapping conversations, particularly if the tool was originally trained on clear, unobstructed audio samples.

A screenshot of Putin’s deepfake was detected as likely to be real, using a detection tool trained for detecting AI images but not for spotting deepfake videos created by swapping people’s faces. Image: Courtesy of Reuters Institute
We tested a detection plugin that was designed to identify fake profile images made by Generative Adversarial Networks (GANs), such as the ones seen in This Person Does Not Exist project. GANs are particularly adept at producing high-quality, domain-specific outputs, such as lifelike faces, in contrast to diffusion models, which excel in generating intricate textures and landscapes. These diffusion models power some of the most talked-about tools of late, including DALL-E, Midjourney, and Stable Diffusion.
Detection tools calibrated to spot synthetic media crafted with GAN technology might not perform as well when faced with content generated or altered by diffusion models. In our testing, the plugin seemed to perform well in identifying results from GAN, likely due to predictable facial features like eyes consistently located at the center of the image.
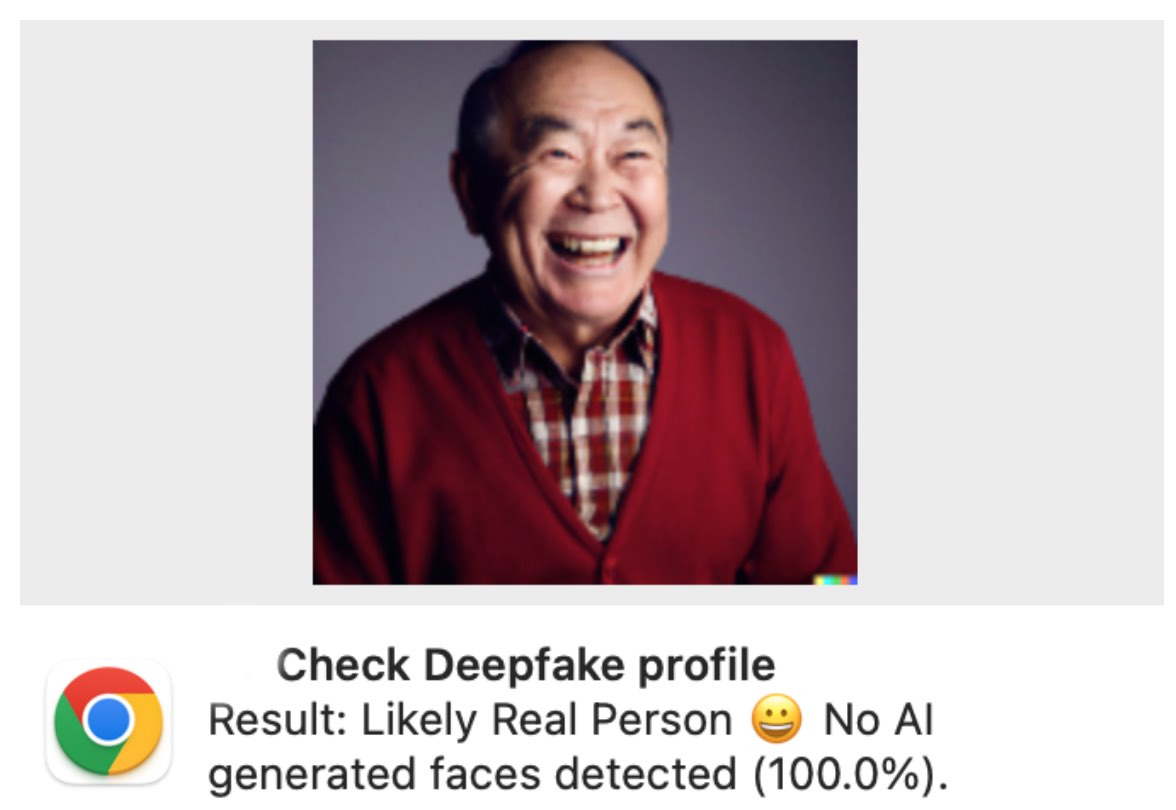
An AI image made with Midjourney was detected to “likely be a real person.” Image: Courtesy of Reuters Institute
An alternative approach to determine whether a piece of media has been generated by AI would be to run it by the classifiers that some companies have made publicly available, such as ElevenLabs. Classifiers developed by companies determine whether a particular piece of content was produced using their tool. This means classifiers are company-specific, and are only useful for signaling whether that company’s tool was used to generate the content. This is important because a negative result just denotes that the specific tool was not employed, but the content may have been generated or edited by another AI tool.
These classifiers face the same detection challenges. For instance, adding music to an audio clip might confuse the classifier and lower the likelihood of the classifier identifying the content as originating from that AI tool. At the moment, no company publicly offers classifiers for images.
How Can You Trick a Detection Tool?
It’s important to keep in mind that tools built to detect whether content is AI-generated or edited may not detect non-AI manipulation.
Editing content: In May 2023, an image showing an explosion in the Pentagon went viral. Though quickly debunked, the image managed to cause a brief panic and even a dip in the stock market. News channels also picked up the story, reporting it as real news.
AI image detection tools were able to identify the image as AI-generated, but after cropping and scaling the right-hand side of the image, the detector suggested the content was real. A similar test was made with one of President Obama’s famous deepfakes. After decreasing the resolution and editing out part of the clip, the result came as “No Deepfake Detected.”
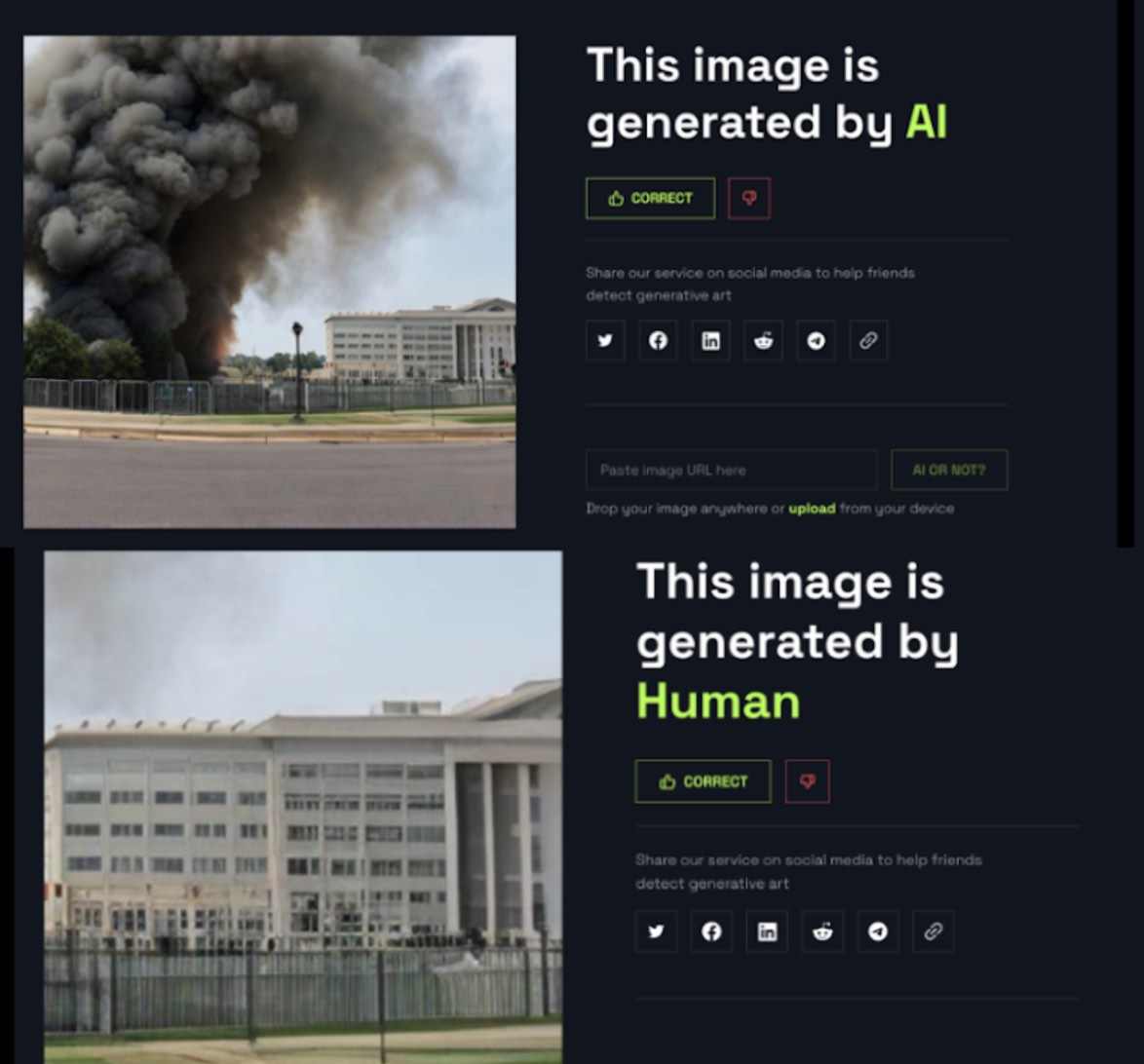
At the top: the image that was shared on social media — and correctly identified as AI-generated. At the bottom: a cut-out of the same image, with the bottom right of the original image cropped and scaled, fooled a classifier tool into thinking it was genuine. Images: Courtesy of Reuters Institute

When we lowered the resolution and edited out the end of this deepfake video of President Obama it was classified as “not a deepfake.” Image: Courtesy of Reuters Institute
Online detection tools might yield inaccurate results with a stripped version of a file (i.e. when information about the file has been removed). This action may not be necessarily malicious or even intentional. For instance, social media platforms may compress a file and eliminate certain metadata during upload.
Even when the training sets may include cropped, blurred, or compressed materials, the act of compressing, cropping, or resizing a file can affect its quality and resolution and impact the detectors–partly because the training materials may not cover all options in which metadata stripping can occur.
Therefore, detection tools may give false results when analyzing such copies. Likewise, when using a recording of an AI-generated audio clip, the quality of the audio decreases, and the original encoded information is lost. For instance, we recorded President Biden’s AI robocall, ran the recorded copy through an audio detection tool, and it was detected as highly likely to be real.
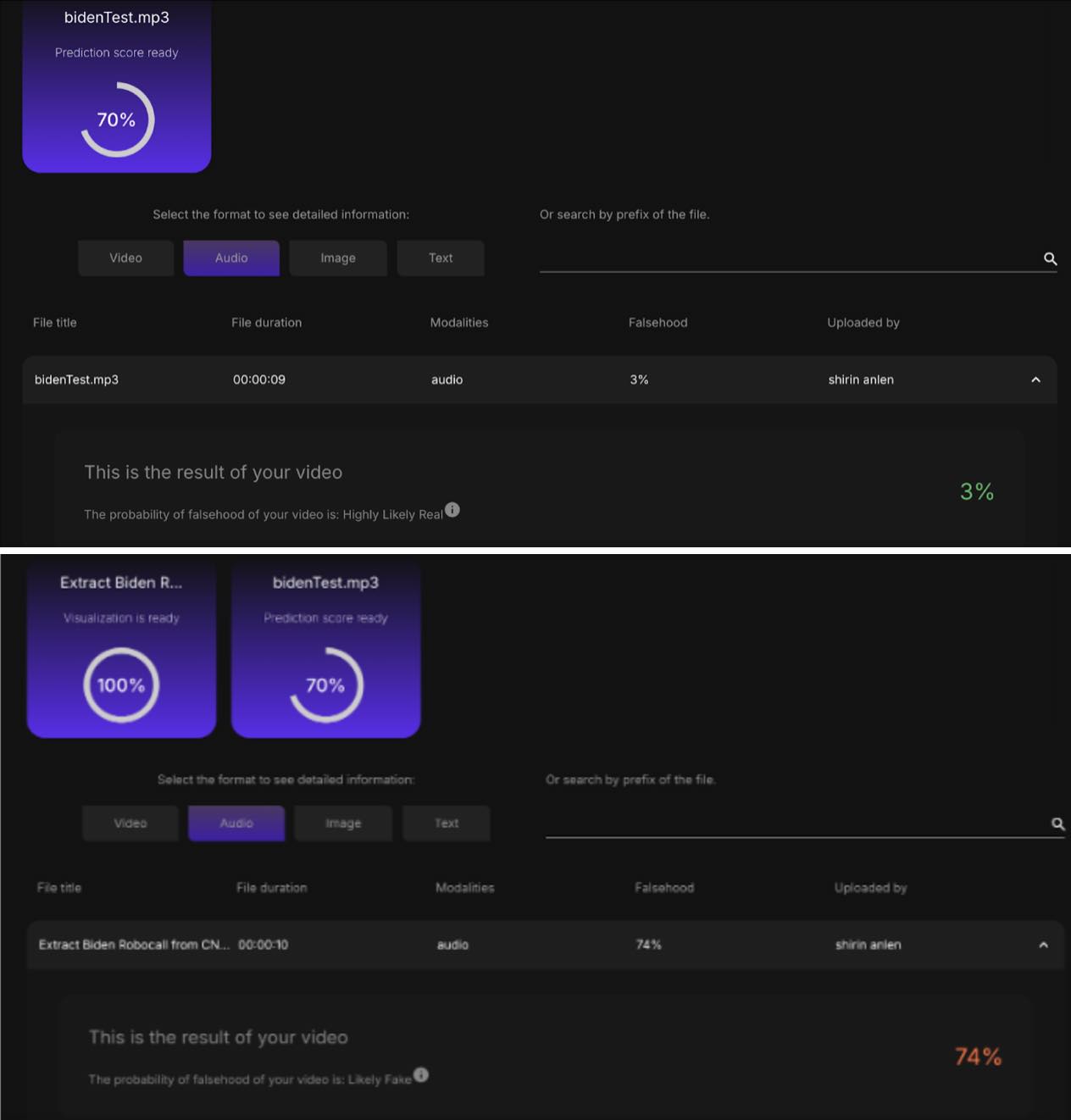
At the top, a recording of President Biden’s robocall was detected as “highly likely real”. By contrast, as the bottom screenshot shows the results with a downloaded version of the file, detected as 74% as “likely to be fake.” Images: Courtesy of Reuters Institute
Similarly, taking a screenshot of an AI-generated image would not contain the same visible and invisible information as the original. We took screenshots from a known case where AI avatars were used to back up a military coup in West Africa. More than half of these screenshots were mistakenly classified as not generated by AI.
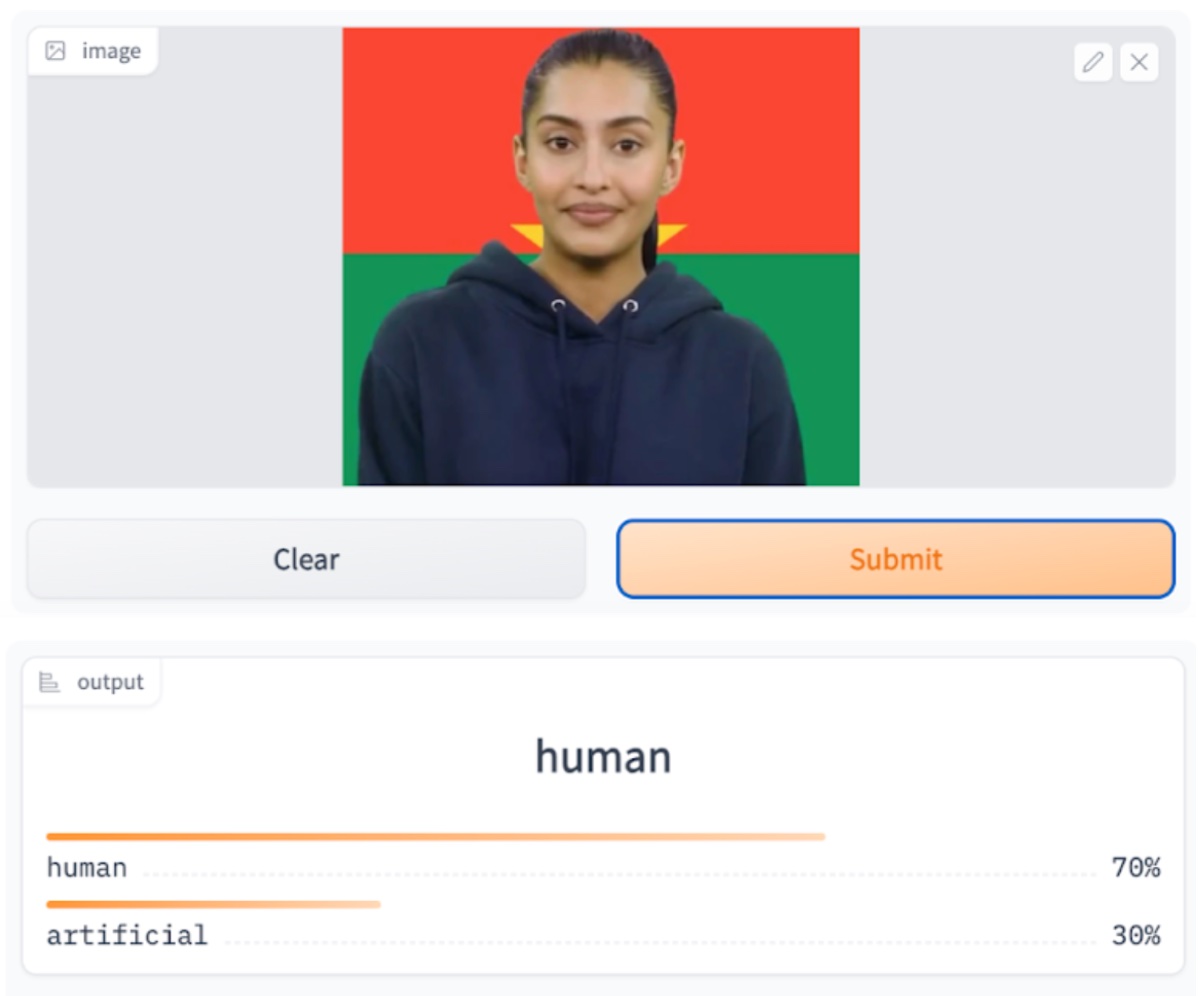
A screenshot with an AI avatar from one of the clips was detected as 70% human. Image: Courtesy of Reuters Institute
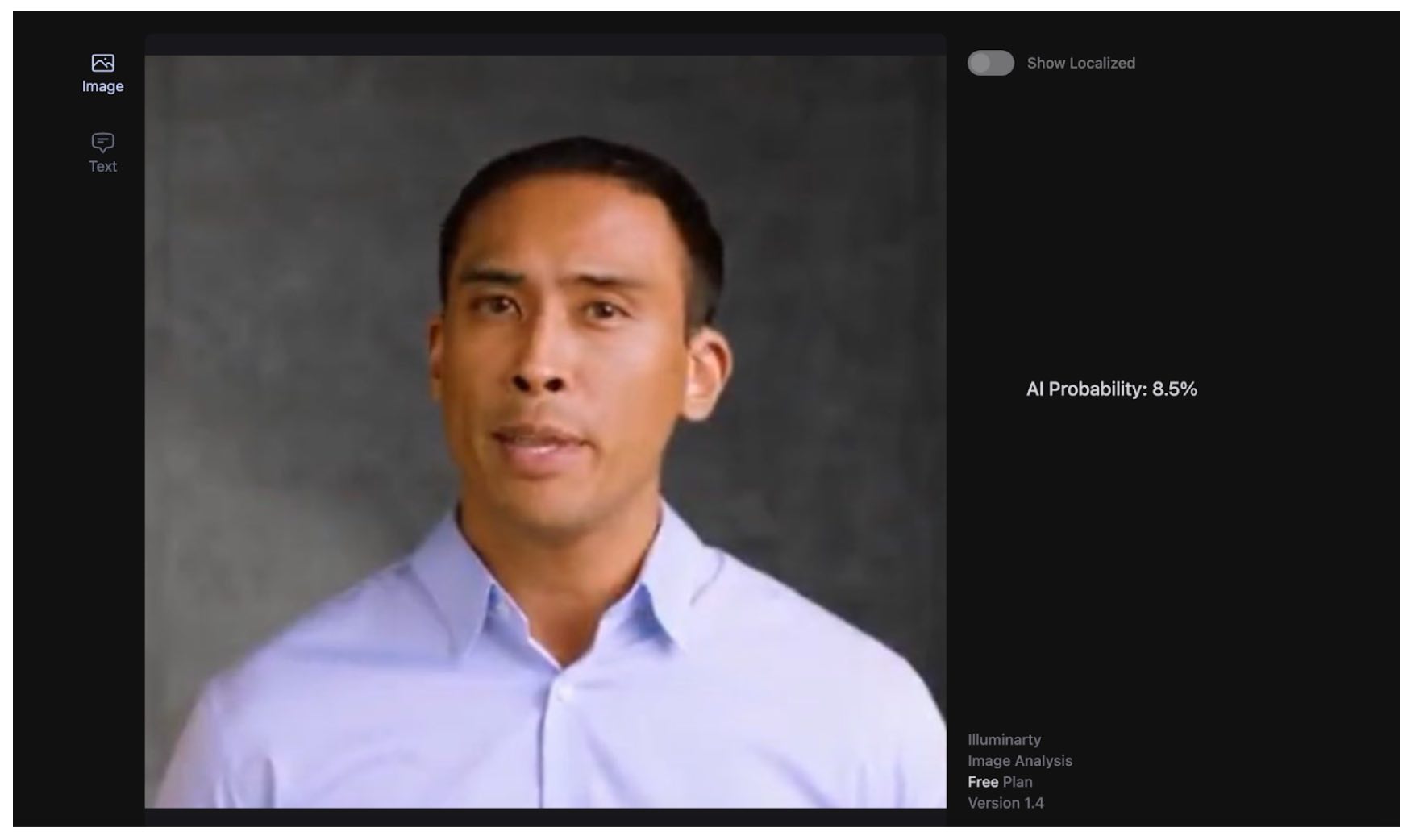
A screenshot with an AI avatar from one of the clips was marked with a 8.5% probability of being AI-generated. Image: Courtesy of Reuters Institute
Can These Tools Detect All Types of AI Generation and Manipulation?
Openly available AI detection software can be fooled by the very AI techniques they are meant to detect. Here is how.
Style prompting: Detection tools are often trained on datasets that were created in a controlled lab environment, where the content is clear and organized. This allows the detection model to learn and identify the necessary features accurately during the training process. In real-world scenarios, though, images may be blurry and out of focus, videos may be shaky and tilted, and audio may be noisy. This can make it challenging for the detection tools to accurately identify and classify real life content.
While detection tools may have been trained with content that imitates what we may find in the “wild,” there are easy ways to confuse a detector.
For instance, we built the blur and compression into the generation through the prompt to test if we could bypass a detector. We used Open AI’s DALL-E 2 to generate realistic images of a number of violent settings, similar to what a bystander may capture on a phone. We gave DALL-E 2 specific instructions to decrease the resolution of the output, and add blur and motion effects. These effects seem to confuse the detection tools, making them believe that the photo was less likely to be AI-generated.
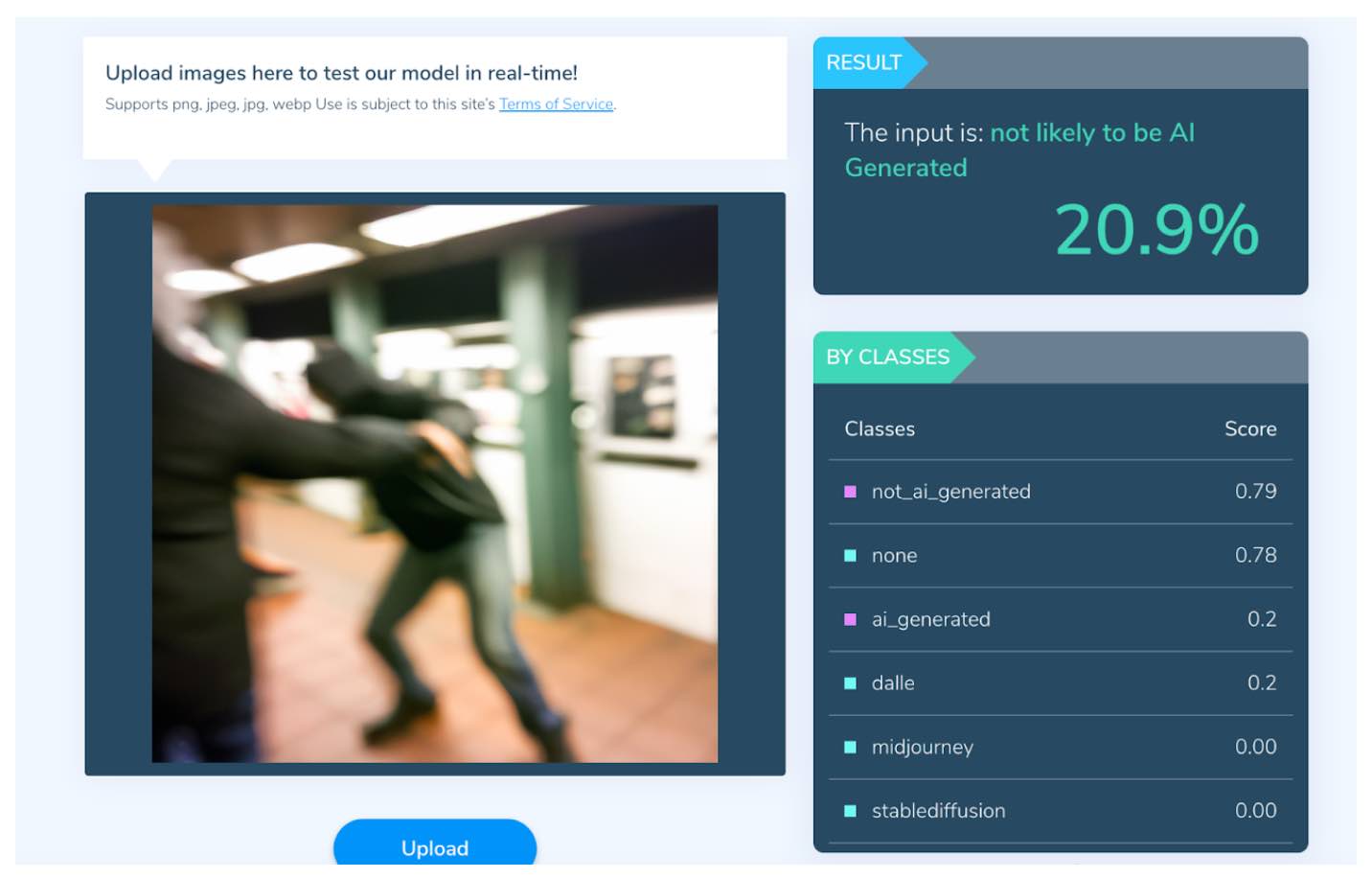
An AI-generated image of a possible attack in a subway station, generated by DALL-E 2, was detected as not likely to be AI-generated. Courtesy of Reuters Institute

AI-generated image of a fake explosion at the US White House, generated by DALL-E 2, and detected with low confidence to be AI-generated. Image: Courtesy of Reuters Institute
Advanced editing: Text-to-image tools have opened the door to easy editing techniques that allow users to edit elements within the frame of an image, or to add new details outside the frame, regardless of the image being real or AI-generated in its origin. These are also known as “in-painting” and “out-painting” techniques, respectively. When they are applied to real images, online tools seem to fail at detecting these manipulations. In this in-paint example, we substituted a car for a tank in a real image of Ukrainian refugees, using DALL-E 2 text-to-image editing. The new image with the tank came back as “not likely to be AI-generated.”
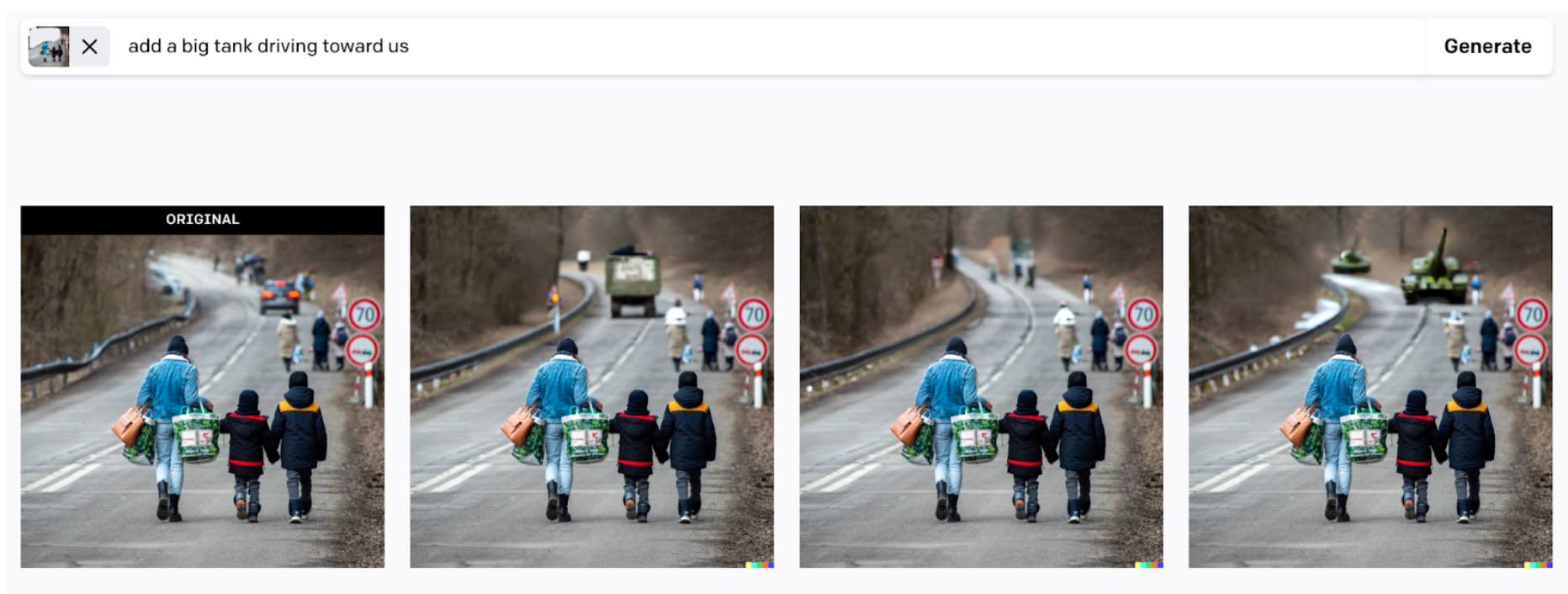
In-painting example using a real image showing Ukrainian refugees, adding a tank with DALL-E 2. Image: Courtesy of Reuters Institute, original photograph by Peter Lazar/AFP/Getty Images.

The image with the in-painted tank was detected as “likely not to be AI-generated.” Image: Courtesy of Reuters Institute, original photograph by Peter Lazar/AFP/Getty Images.
As an example of out-painting, we took a real image from the Israel-Hamas war and used DALL-E 2 to add the extra context of “smoke.” DALL-E 2 also extended the buildings in the image. The final output was detected as real.
Outpaint example using a real image showing soldiers in the Gaza-Israel war. Image: Courtesy of Reuters Institute, original photograph by Reuters

This tool detected the out-painted output as “likely human.” Courtesy of Reuters Institute
So What Can Journalists, Fact-checkers, and Researchers Do?
Although this piece identifies some of the limitations of online AI detection tools, they can still be a valuable resource as part of the verification process or an investigative methodology, as long as they are used thoughtfully.
It is essential to approach them with a critical eye, recognizing that their efficacy is contingent upon the data and algorithms they were built upon. Additionally, it is important to keep in mind that, with the exception of audio content, most of the footage we see online tends to be mis-contextualized material (circulated with the wrong date, time, or location) or images edited with software that has already been available for a few years.
The biggest threat brought by audiovisual generative AI is that it has opened up the possibility of plausible deniability, by which anything can be claimed to be a deepfake.
Detection tools should be used with caution and skepticism, and it is always important to research and understand how a tool was developed, but this information may be difficult to obtain.
As technology advances, previously effective algorithms begin to lose their edge, necessitating continuous innovation and adaptation to stay ahead. As soon as one method becomes obsolete, new, more sophisticated techniques must be developed to counteract the latest advancements in synthetic media creation. While more holistic responses to the threats of synthetic media are addressed across the information pipeline, it is essential for those working on verification to stay abreast of both generation and detection techniques.
It is also key to consider that AI technology is increasingly incorporated across all kinds of software, from phone cameras to social media apps; and it is also involved in multiple stages of production, from stabilizing cameras and applying filters, to erasing unwanted objects and subjects from the frame.
AI may not necessarily generate new content, but it can be applied to affect a specific region of the content, and a specific keyframe and time. The complex and wide range of manipulations compounds the challenges of detection. New tools, versions, and features are constantly being developed, leading to questions about how well, and how frequently detectors are being updated and maintained.
Security and ethical considerations matter too. Given the uncertainty surrounding the storage and use of analyzed content by these platforms, it is vital to weigh the privacy and security risks, especially when dealing with material that could impact the privacy and safety of real individuals depicted in an image, audio, or video.
In light of these considerations, when disseminating findings, it is essential to clearly articulate the verification process, including the tools used, their known limitations, and the interpretation of their confidence levels. This openness not only bolsters the credibility of the verification but also educates the audience on the complexities of detecting synthetic media. For content bearing a visible watermark of the tool that was used to generate it, consulting the tool’s proprietary classifier can offer additional insights. However, remember that a classifier’s confirmation only verifies the use of its respective tool, not the absence of manipulation by other AI technologies.
Editor’s Note: This story was originally published by the Reuters Institute and is reposted here with permission.
shirin anlen is an award-winning creative technologist, researcher, and artist based in New York. Her work explores the societal implications of emerging technology, with a focus on internet platforms and artificial intelligence. At WITNESS, she is part of the Technology, Threats, and Opportunities program, investigating deepfakes, media manipulation, content authenticity, and cryptography practices in the space of human rights violations. She is a research fellow at the MIT Open Documentary Lab, a member of Women+ Art AI, and holds an MFA in Cinema and Television from Tel Aviv University, where she majored in interactive documentary making.
Raquel Vázquez Llorente is a lawyer specializing in audiovisual media in conflict and human rights crises. At WITNESS, she leads a team that critically examines the impact of emerging technologies, especially generative AI and deepfakes, on our trust in audiovisual media. Her policy portfolio also focuses on the operational and regulatory challenges associated with the retention and disclosure of social media content that may be probative of international crimes. She serves on the Board of The Guardian Foundation, and on the Advisory Board of TRUE, a project that studies the impact of deepfakes on trust in user-generated evidence in accountability processes for human rights violations. She is also a member of PAI (Partnership on AI) Policy Steering Committee, a body considering pressing questions in AI governance. She holds an MSc in International Strategy and Diplomacy from the London School of Economics and Political Science (LSE), and an Advanced Degree in Law and Business Administration from Universidad Carlos III de Madrid.